期刊论文
我院教师李伟就随机缺失中的多稳健估计问题在《Biometrika》发表论文
时间:2021-03-07
论文题目
Demystifying a class of multiply robust estimators
作者介绍
李伟,中国人民大学统计学院生物统计与流行病学教师,应用统计科学研究中心研究员,北京大学数学科学学院博士。主要研究领域为因果推断、缺失数据、机器学习及高维统计等。目前已在包括Biometrika, Journal of Econometrics, Scandinavian Journal of Statistics, Statistics in Medicine等国际著名统计期刊上发表多篇学术论文。主持一项中国博士后科学基金项目,参与完成多项国家自然科学基金项目。
内容摘要
该研究主要讨论响应变量数据随机缺失时其总体均值的稳健估计问题。许多文献针对这一问题提出了多稳健估计量,即允许倾向性评分和响应变量回归分别有多个候选模型,而当这若干模型有一个被正确指定时,所提估计量便是相合的。我们在本文中指出这些多稳健估计量其实是双稳健估计量的特殊情形。基于此种发现,我们利用模型混合的思想,分别把倾向性评分的多个模型和响应变量的多个模型加权整合成一个倾向性评分和一个响应变量回归模型,然后代入已有的双稳健估计量,构造一个新的估计量。我们证明了所提估计量也具有多稳健性质。相比以往学者提出的多稳健估计方法,我们则在非参数一般模型下讨论了所提估计量的相合性和渐近正态性,大大拓展了该类方法的理论框架和应用范围。
英文摘要
For estimating the population mean of a response variable subject to ignorable missingness, a new class of methods, called multiply robust procedures, has been proposed. The advantage of multiply robust procedures over the traditional doubly robust methods is that they permit the use of multiple candidate models for both the propensity score and the outcome regression, and they are consistent if any one of the multiple models is correctly specified, a property termed multiple robustness. This paper shows that, somewhat surprisingly, multiply robust estimators are special cases of doubly robust estimators, where the final propensity score and outcome regression models are certain combinations of the candidate models. To further improve model specifications in the doubly robust estimators, we adapt a model mixing procedure as an alternative method for combining multiple candidate models. We show that multiple robustness and asymptotic normality can also be achieved by our mixing-based doubly robust estimator. Moreover, our estimator and its theoretical properties are not confined to parametricmodels. Numerical examples demonstrate that the proposed estimator is comparable to and can even outperform existing multiply robust estimators.
发表页面
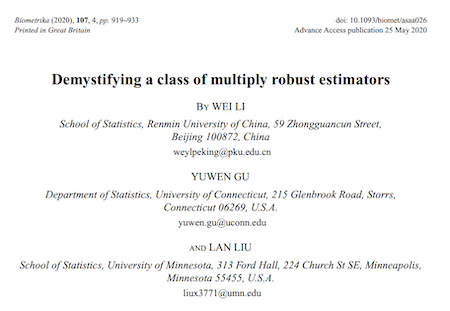